Hello! I am a first year PhD student at Washington University in St. Louis majoring in Computer Science. I’m fortunately advised by Prof. Yixin Chen. Prior to this, I earned my master’s degree at Zhejiang University, fortunately advised by Prof. Yang Yang, and my bachelor’s degree at Huazhong University of Science and Technology.
My research interests primarily include Machine Learning and Data Mining, with a particular focus on time series and graph, and it’s applications in healthcare, social media, etc. During my tenure in Alibaba, I also developed interests in LLMs and Foundation Models (in time series and graph).
🔥 News
- 2024.12: 🧑💻🧑💻 After reaching an agreement with cooperated hospital and company, we can finally public the code for MBrain. Check the code here
.
- 2024.08: ✨✨ I started pursuing my PhD Degree in WashU.
📝 Publications
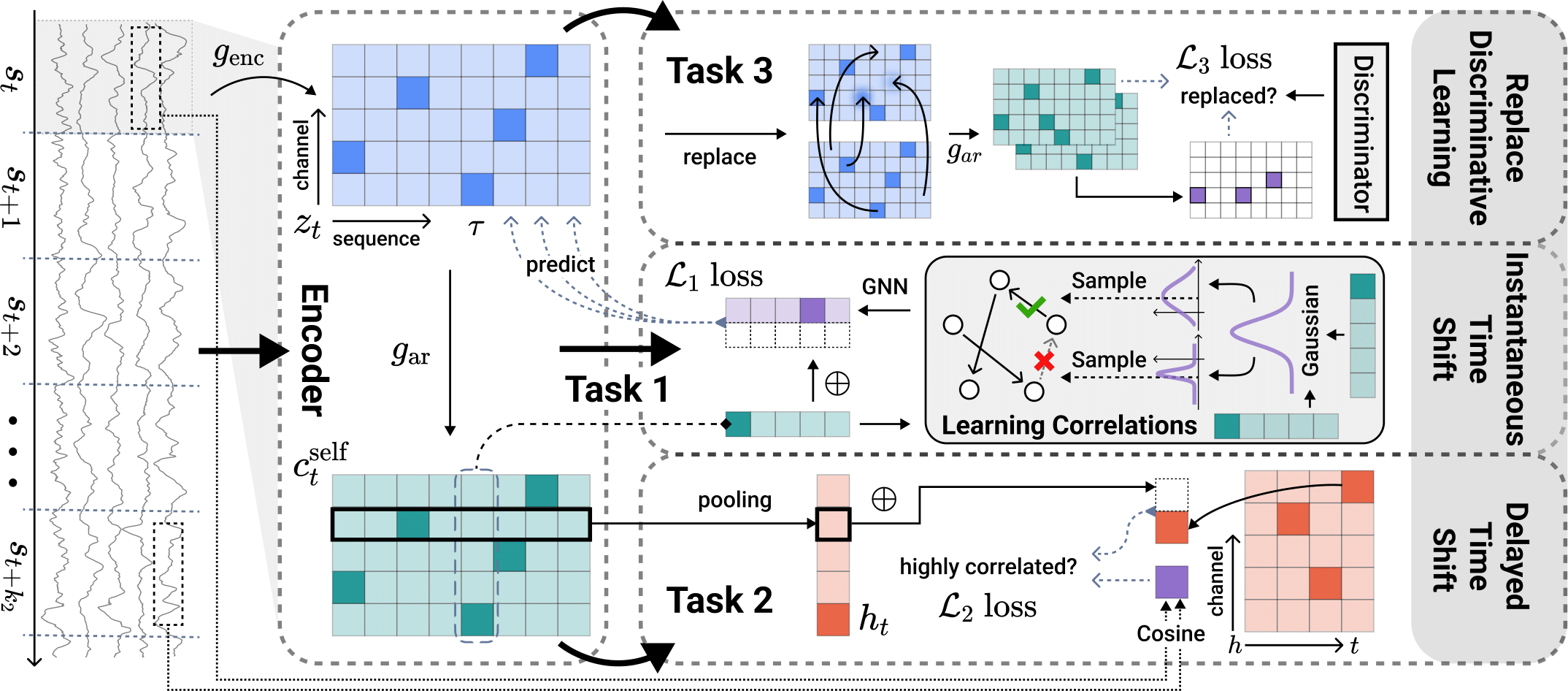
MBrain: A Multi-channel Self-Supervised Learning Framework for Brain Signals
Donghong Cai, Junru Chen, Yang Yang, Teng Liu, Yafeng Li
MBrain is the first generalized self-supervised learning framework, which can be applied to pre-train both EEG and SEEG signals.
📖 Educations
- 2024.08 - present, PhD, Washington University in St. Louis, Saint Louis.
- 2020.09 - 2023.03, Master, Zhejiang University, Hangzhou.
- 2016.09 - 2020.06, Undergraduate, Huazhong University of Science and Technology, Wuhan.
💻 Internships and Professional Experiences
- 2023.04 - 2024.07, Machine Learning Engineer, Alibaba, China.